from dsc80_utils import *
Announcements 📣¶
- Lab 2 due tomorrow, Wed, Jan 22.
- Project 1 is due this Friday, Jan 24.
Agenda 📆¶
- Dataset overview.
- Introduction to
plotly
. - Exploratory data analysis and feature types.
- Data cleaning.
- Data quality checks.
- Missing values.
- Transformations and timestamps.
- Modifying structure.
- Investigating student-submitted questions!
Dataset overview¶
San Diego food safety¶
From this article (archive link):
In the last three years, one third of San Diego County restaurants have had at least one major food safety violation.
99% Of San Diego Restaurants Earn ‘A' Grades, Bringing Usefulness of System Into Question¶
From this article (archive link):
Food held at unsafe temperatures. Employees not washing their hands. Dirty countertops. Vermin in the kitchen. An expired restaurant permit.
Restaurant inspectors for San Diego County found these violations during a routine health inspection of a diner in La Mesa in November 2016. Despite the violations, the restaurant was awarded a score of 90 out of 100, the lowest possible score to achieve an ‘A’ grade.
The data¶
- We downloaded the data about the 1000 restaurants closest to UCSD from here.
- We had to download the data as JSON files, then process it into DataFrames. You'll learn how to do this soon!
- Until now, you've (largely) been presented with CSV files that
pd.read_csv
could load without any issues. - But there are many different formats and possible issues when loading data in from files.
- See Chapter 8 of Learning DS for more.
- Until now, you've (largely) been presented with CSV files that
rest_path = Path('data') / 'restaurants.csv'
insp_path = Path('data') / 'inspections.csv'
viol_path = Path('data') / 'violations.csv'
rest = pd.read_csv(rest_path)
insp = pd.read_csv(insp_path)
viol = pd.read_csv(viol_path)
Question 🤔 (Answer at dsc80.com/q)
Code: dfs
The first article said that one third of restaurants had at least one major safety violation.
Which DataFrames and columns seem most useful to verify this?
rest.head(2)
rest.columns
insp.head(2)
insp.columns
viol.head(2)
viol.columns
Introduction to plotly
¶
plotly
¶
- We've used
plotly
in lecture briefly, and you even have to use it in Project 1 Question 13, but we haven't yet discussed it formally.
- It's a visualization library that enables interactive visualizations.
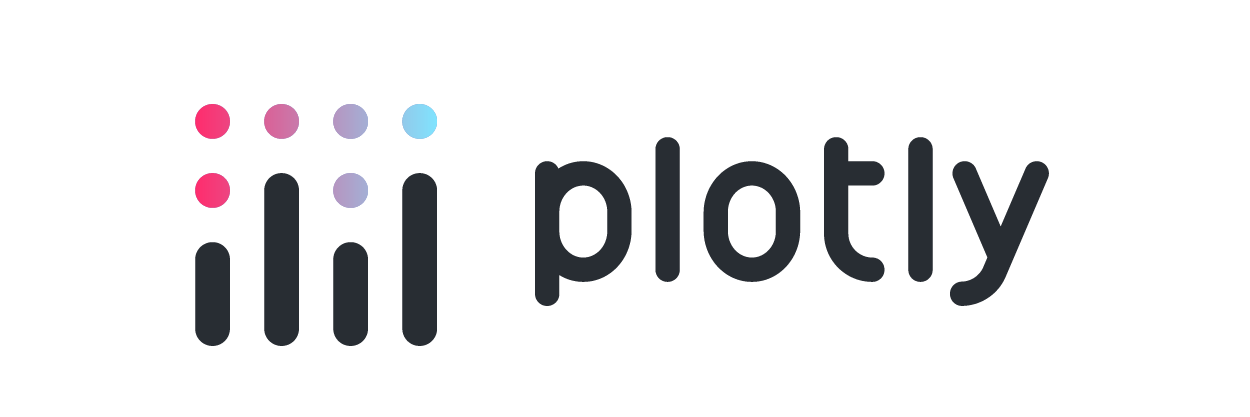
Using plotly
¶
There are a few ways we can use plotly
:
- Using the
plotly.express
syntax.plotly
is very flexible, but it can be verbose;plotly.express
allows us to make plots quickly.- See the documentation here – it's very rich (there are good examples for almost everything).
- By setting
pandas
plotting backend to'plotly'
(by default, it's'matplotlib'
) and using the DataFrameplot
method.- The DataFrame
plot
method is how you created plots in DSC 10!
- The DataFrame
For now, we'll use plotly.express
syntax; we've imported it in the dsc80_utils.py
file that we import at the top of each lecture notebook.
Initial plots¶
First, let's look at the distribution of inspection 'score'
s:
fig = px.histogram(insp['score'])
fig
How about the distribution of average inspection 'score'
per 'grade'
?
scores = (
insp[['grade', 'score']]
.dropna()
.groupby('grade')
.mean()
.reset_index()
)
# x= and y= are columns of scores. Convenient!
px.bar(scores, x='grade', y='score')
# Same as the above!
scores.plot(kind='bar', x='grade', y='score')
Exploratory data analysis and feature types¶
Exploratory data analysis (EDA)¶
Historically, data analysis was dominated by formal statistics, including tools like confidence intervals, hypothesis tests, and statistical modeling.
In 1977, John Tukey defined the term exploratory data analysis, which described a philosophy for proceeding about data analysis:
Exploratory data analysis is actively incisive, rather than passively descriptive, with real emphasis on the discovery of the unexpected.
- Practically, EDA involves, among other things, computing summary statistics and drawing plots to understand the nature of the data at hand.
The greatest gains from data come from surprises… The unexpected is best brought to our attention by pictures.
Different feature types¶
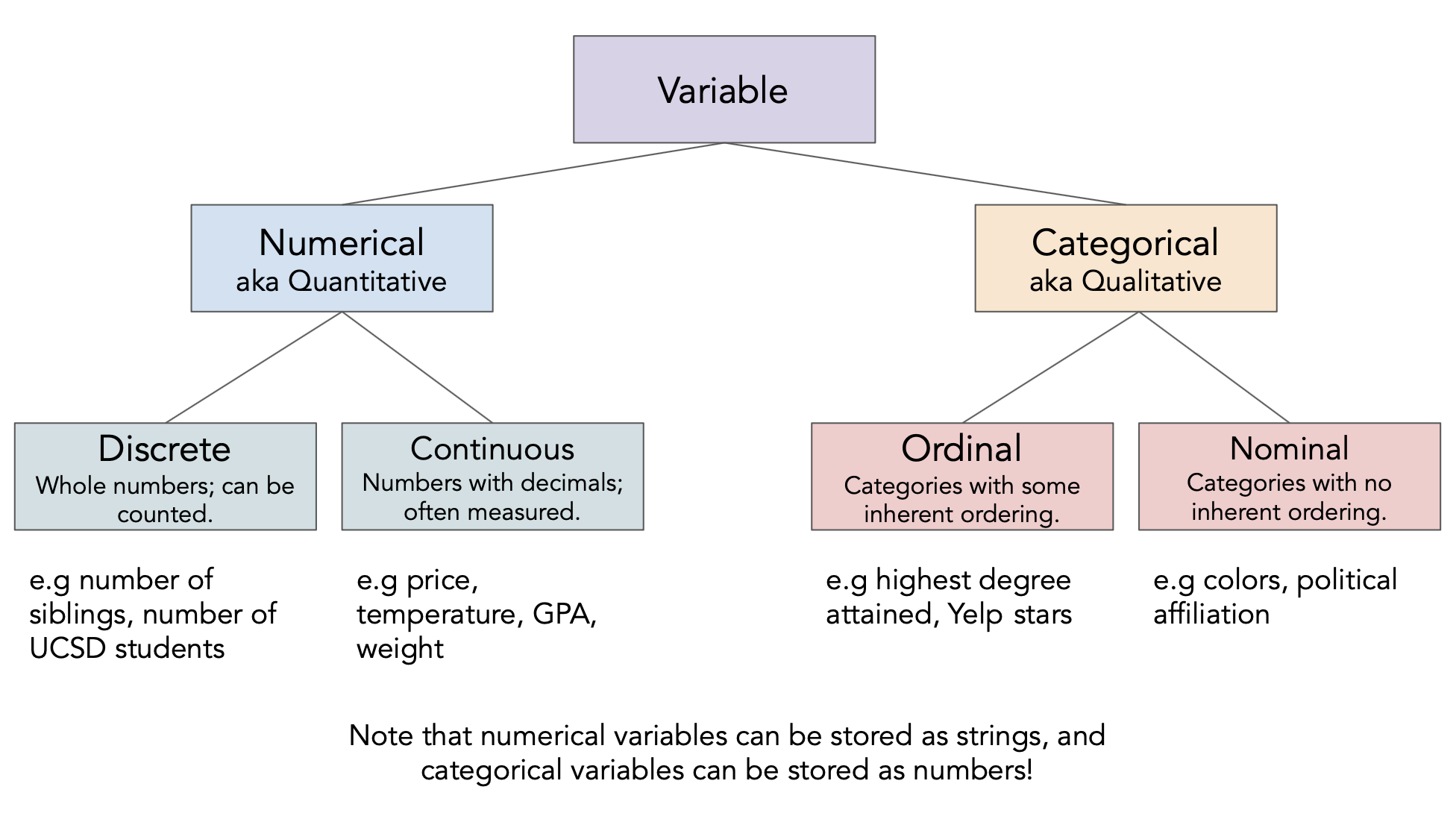
Question 🤔 (Answer at dsc80.com/q)
Code: types
Determine the feature type of each of the following variables.
insp['score']
insp['grade']
viol['violation_accela']
viol['major_violation']
rest['business_id']
rest['opened_date']
# Your code goes here.
Feature types vs. data types¶
The data type
pandas
uses is not the same as the "data type" we talked about just now!- There's a difference between feature type and computational data type.
Take care when the two don't match up very well!
# pandas stores these as ints, but they're actually nominal.
rest['business_id']
# pandas stores these as strings, but they're actually numeric.
rest['opened_date']
Data cleaning¶
Four pillars of data cleaning¶
When loading in a dataset, to clean the data – that is, to prepare it for further analysis – we will:
Perform data quality checks.
Identify and handle missing values.
Perform transformations, including converting time series data to timestamps.
Modify structure as necessary.
Data cleaning: Data quality checks¶
Data quality checks¶
We often start an analysis by checking the quality of the data.
- Scope: Do the data match your understanding of the population?
- Measurements and values: Are the values reasonable?
- Relationships: Are related features in agreement?
- Analysis: Which features might be useful in a future analysis?
Scope¶
Do the data match your understanding of the population?
We were told that we're only looking at the 1000 restaurants closest to UCSD, so the restaurants in rest
should agree with that.
rest.sample(5)
Measurements and values¶
Are the values reasonable?
Do the values in the 'grade'
column match what we'd expect grades to look like?
insp['grade'].value_counts()
What kinds of information does the insp
DataFrame hold?
insp.info()
What's going on in the 'address'
column of rest
?
# Are there multiple restaurants with the same address?
rest['address'].value_counts()
# Keeps all rows with duplicate addresses.
(
rest
.groupby('address')
.filter(lambda df: df.shape[0] >= 2)
.sort_values('address')
)
# Does the same thing as above!
(
rest[rest.duplicated(subset=['address'], keep=False)]
.sort_values('address')
)
Relationships¶
Are related features in agreement?
Do the 'address'
es and 'zip'
codes in rest
match?
rest[['address', 'zip']]
What about the 'score'
s and 'grade'
s in insp
?
insp[['score', 'grade']]
Analysis¶
Which features might be useful in a future analysis?
We're most interested in:
- These columns in the
rest
DataFrame:'business_id'
,'name'
,'address'
,'zip'
, and'opened_date'
. - These columns in the
insp
DataFrame:'business_id'
,'inspection_id'
,'score'
,'grade'
,'completed_date'
, and'status'
. - These columns in the
viol
DataFrame:'inspection_id'
,'violation'
,'major_violation'
,'violation_text'
, and'violation_accela'
.
- These columns in the
Also, let's rename a few columns to make them easier to work with.
💡 Pro-Tip: Using pipe
¶
When we manipulate DataFrames, it's best to define individual functions for each step, then use the pipe
method to chain them all together.
The pipe
DataFrame method takes in a function, which itself takes in a DataFrame and returns a DataFrame.
- In practice, we would add functions one by one to the top of a notebook, then
pipe
them all. - For today, will keep re-running
pipe
to show data cleaning process.
def subset_rest(rest):
return rest[['business_id', 'name', 'address', 'zip', 'opened_date']]
rest = (
pd.read_csv(rest_path)
.pipe(subset_rest)
)
rest
# Same as the above – but the above makes it easier to chain more .pipe calls afterwards.
subset_rest(pd.read_csv(rest_path))
Let's use pipe
to keep (and rename) the subset of the columns we care about in the other two DataFrames as well.
def subset_insp(insp):
return (
insp[['business_id', 'inspection_id', 'score', 'grade', 'completed_date', 'status']]
.rename(columns={'completed_date': 'date'})
)
insp = (
pd.read_csv(insp_path)
.pipe(subset_insp)
)
def subset_viol(viol):
return (
viol[['inspection_id', 'violation', 'major_violation', 'violation_accela']]
.rename(columns={'violation': 'kind',
'major_violation': 'is_major',
'violation_accela': 'violation'})
)
viol = (
pd.read_csv(viol_path)
.pipe(subset_viol)
)
Combining the restaurant data¶
Let's join all three DataFrames together so that we have all the data in a single DataFrame.
def merge_all_restaurant_data():
return (
rest
.merge(insp, on='business_id', how='left')
.merge(viol, on='inspection_id', how='left')
)
df = merge_all_restaurant_data()
df
Question 🤔 (Answer at dsc80.com/q)
Code: lefts
Why should the function above use two left joins? What would go wrong if we used other kinds of joins?
Data cleaning: Missing values¶
Missing values¶
Next, it's important to check for and handle missing values, as they can have a big effect on your analysis.
insp[['score', 'grade']]
# The proportion of values in each column that are missing.
insp.isna().mean()
# Why are there null values here?
# insp['inspection_id'] and viol['inspection_id'] don't have any null values...
df[df['inspection_id'].isna()]
There are many ways of handling missing values, which we'll cover in an entire lecture next week. But a good first step is to check how many there are!
Data cleaning: Transformations and timestamps¶
Transformations and timestamps¶
From last class:
A transformation results from performing some operation on every element in a sequence, e.g. a Series.
It's often useful to look at ways of transforming your data to make it easier to work with.
Type conversions (e.g. changing the string
"$2.99"
to the number2.99
).Unit conversion (e.g. feet to meters).
Extraction (Getting
'vermin'
out of'Vermin Violation Recorded on 10/10/2023'
).
Creating timestamps¶
Most commonly, we'll parse dates into pd.Timestamp
objects.
# Look at the dtype!
insp['date']
# This magical string tells Python what format the date is in.
# For more info: https://docs.python.org/3/library/datetime.html#strftime-and-strptime-behavior
date_format = '%Y-%m-%d'
pd.to_datetime(insp['date'], format=date_format)
# Another advantage of defining functions is that we can reuse this function
# for the 'opened_date' column in `rest` if we wanted to.
def parse_dates(insp, col):
date_format = '%Y-%m-%d'
dates = pd.to_datetime(insp[col], format=date_format)
return insp.assign(**{col: dates})
insp = (
pd.read_csv(insp_path)
.pipe(subset_insp)
.pipe(parse_dates, 'date')
)
# We should also remake df, since it depends on insp.
# Note that the new insp is used to create df!
df = merge_all_restaurant_data()
# Look at the dtype now!
df['date']
Working with timestamps¶
- We often want to adjust granularity of timestamps to see overall trends, or seasonality.
- Use the
resample
method inpandas
(documentation).- Think of it like a version of
groupby
, but for timestamps. - For instance,
insp.resample('2W', on='date')
separates every two weeks of data into a different group.
- Think of it like a version of
insp.resample('2W', on='date')['score'].mean()
# Where are those numbers coming from?
insp[
(insp['date'] >= pd.Timestamp('2020-01-05')) &
(insp['date'] < pd.Timestamp('2020-01-19'))
]['score']
(insp.resample('2W', on='date')
.size()
.plot(title='Number of Inspections Over Time')
)
The .dt
accessor¶
Like with Series of strings, pandas
has a .dt
accessor for properties of timestamps (documentation).
insp['date']
insp['date'].dt.day
insp['date'].dt.dayofweek
dow_counts = insp['date'].dt.dayofweek.value_counts()
fig = px.bar(dow_counts)
fig.update_xaxes(tickvals=np.arange(7), ticktext=['Mon', 'Tues', 'Wed', 'Thurs', 'Fri', 'Sat', 'Sun'])
Data cleaning: Modifying structure¶
Reshaping DataFrames¶
We often reshape the DataFrame's structure to make it more convenient for analysis. For example, we can:
Simplify structure by removing columns or taking a set of rows for a particular period of time or geographic area.
- We already did this!
Adjust granularity by aggregating rows together.
- To do this, use
groupby
(orresample
, if working with timestamps).
- To do this, use
Reshape structure, most commonly by using the DataFrame
melt
method to un-pivot a dataframe.
Using melt
¶
- The
melt
method is common enough that we'll give it a special mention. - We'll often encounter pivot tables (esp. from government data), which we call wide data.
- The methods we've introduced work better with long-form data, or tidy data.
- To go from wide to long,
melt
.
Example usage of melt
¶
wide_example = pd.DataFrame({
'Year': [2001, 2002],
'Jan': [10, 130],
'Feb': [20, 200],
'Mar': [30, 340]
}).set_index('Year')
wide_example
wide_example.melt(ignore_index=False)
Exploration¶
Question 🤔 (Answer at dsc80.com/q)
Code: qs
What questions do you want me to try and answer with the data? I'll start with a single pre-prepared question, and then answer student questions until we run out of time.
Example question: Can we rank restaurants by their number of violations? How about separately for each zip code?¶
And why would we want to do that? 🤔
Summary, next time¶
Summary¶
- Data cleaning is a necessary starting step in data analysis. There are four pillars of data cleaning:
- Quality checks.
- Missing values.
- Transformations and timestamps.
- Modifying structure.
- Approach EDA with an open mind, and draw lots of visualizations.
Next time¶
Hypothesis and permutation testing. Some of this will be DSC 10 review, but we'll also push further!